In the intricate world of financial institutions, the cornerstone of maintaining integrity and security lies in the adept management of risks. These risks, ranging from fraud to anti-money laundering (AML) compliance, are dynamic forces, constantly evolving in response to a multitude of factors such as technological advancements, changing economic conditions, and novel fraudulent practices. To navigate this complex landscape, financial institutions have increasingly relied on sophisticated risk assessment models. However, a critical aspect often overlooked in this scenario is the phenomenon of model drift.
At its core, model drift represents a gradual but significant change in the effectiveness of risk assessment models over time. This change is not just a statistical or technological issue; it's a real-world challenge that can have substantial implications on an institution's ability to detect and prevent fraud, comply with AML regulations, and safeguard its operations against a myriad of financial crimes. As these risk models form the backbone of strategic decision-making, their degradation – often subtle and unnoticed – can lead to substantial vulnerabilities.
The reasons behind the neglect of model drift are as varied as they are impactful. From a false sense of security instilled by initially successful models to the daunting task of continuously updating and monitoring these complex systems, the factors contributing to this oversight are numerous. Additionally, external elements like rapidly evolving market conditions, changing criminal tactics, and regulatory amendments contribute to the alteration of the risk landscape, further exacerbating the problem.
This oversight is particularly concerning considering the stakes involved. Ineffective risk models can result in failed fraud detection, erroneous risk assessments, and inefficient allocation of resources, not to mention the reputational damage and regulatory penalties that can arise from these failures. Thus, understanding and addressing model drift is not just a technical necessity but a strategic imperative for financial institutions.
As we delve deeper into this topic, we aim to uncover the nuances of model drift in risk assessment. We'll explore its types, causes, impacts, and, most importantly, the strategies to mitigate it. The goal is to shed light on this often-ignored aspect of risk management and provide insights that can help institutions fortify their defenses against an ever-changing landscape of financial risks. The journey through this discussion is not just about understanding a concept but about equipping oneself with the knowledge to stay ahead in a world where risk is a constant, but its nature is anything but.
Defining model drift
Model drift, in the context of risk assessment in financial institutions, is a subtle yet profound concept that necessitates a clear understanding. It refers to the phenomenon where the predictive accuracy and reliability of a risk assessment model gradually diminish over time. This degradation occurs as the real-world data and scenarios, which the model is designed to analyze and predict, evolve in ways that were not anticipated when the model was originally developed. To fully grasp this concept, it’s essential to break it down into its fundamental components.
1. What constitutes a model in risk assessment?
A model, in this sense, is an algorithmic tool that financial institutions use to predict risk. It can range from simple statistical models to complex machine learning algorithms. These models analyze vast amounts of data to identify patterns, anomalies, and potential risks related to fraud, money laundering, and other financial crimes.
2. The nature of drift in these models:
The drift in these models isn't typically abrupt; rather, it's a gradual process where the model's predictions become less accurate over time. This decline can be so subtle that it often goes unnoticed until the model's effectiveness has significantly decreased.
3. Types of model drift:
- Data drift: Occurs when the statistical properties of the input data change. For example, a change in customer behavior or transaction patterns can lead to data drift.
- Concept drift: This happens when the relationship between the input data and the output variable changes. For instance, new types of financial fraud can alter the underlying patterns that a fraud detection model was trained to recognize.
- Population drift: Refers to changes in the population that the model is analyzing. As the customer base of a financial institution evolves, the model may no longer accurately represent the risk profile of the new clientele.
4. Why model drift occurs:
- Model drift is an inevitable consequence of the dynamic nature of the financial world. Changes in economic conditions, customer behavior, market trends, regulatory environments, and criminal tactics can all contribute to model drift.
- Technological advancements also play a role. As new data sources and analytical tools become available, the way we understand and predict risk evolves, potentially rendering existing models less effective.
Understanding model drift is crucial for financial institutions as it directly impacts their ability to manage risk effectively. Ignoring model drift can lead to a false sense of security, where outdated models give the illusion of control and accuracy, potentially exposing the institution to unanticipated risks. By acknowledging and understanding the concept of model drift, financial institutions can take proactive steps to ensure their risk assessment models remain accurate, relevant, and effective in the ever-changing landscape of financial risks. This understanding forms the foundation for developing strategies to monitor, detect, and counteract model drift, thereby maintaining the integrity and reliability of risk management processes.
The overlooked aspect: Continuous monitoring and updating
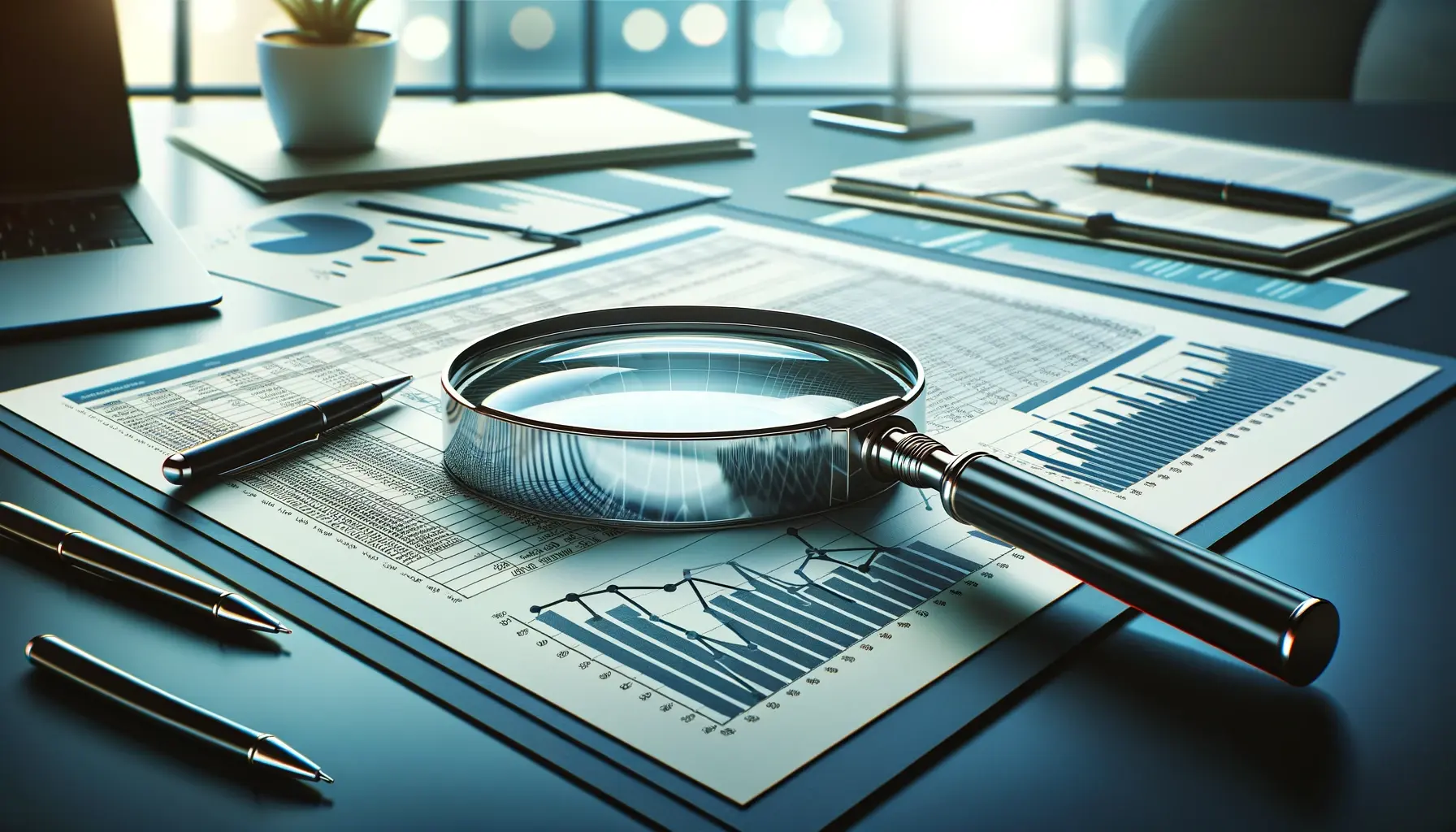
In the realm of risk assessment, the crucial but often overlooked aspect is the continuous monitoring and updating of risk models. This ongoing process is essential for maintaining the relevance and accuracy of these models in the face of the ever-evolving financial landscape. However, due to various reasons, this critical practice is frequently neglected, leading to significant consequences.
1. The importance of continuous monitoring:
Continuous monitoring involves regularly evaluating the performance of risk assessment models to ensure they accurately reflect current data and risk scenarios. This process is vital because it helps identify the onset of model drift before it significantly impacts the model's predictive capabilities. Without consistent monitoring, there is a risk of relying on outdated models, which can lead to incorrect risk assessments, missed fraudulent activities, and compliance issues.
2. Challenges in model updating:
Updating a risk model isn't a one-time task but an ongoing process that requires resources, expertise, and commitment. It involves retraining models with new data, adjusting algorithms to reflect current trends, and re-evaluating the entire risk assessment framework. One of the main challenges here is resource allocation. Continuous updating requires dedicated personnel and technological resources, which can be a significant investment for many institutions.
3. Technological and skill-based constraints:
The rapid advancement of technology in data analytics and machine learning presents both an opportunity and a challenge. Keeping up with these advancements necessitates specialized skills and understanding, which might not always be available in-house. Additionally, integrating new technologies into existing systems can be complex and time-consuming.
4. The consequences of neglect:
Ignoring the need for continuous monitoring and updating can lead to a false sense of security. Financial institutions might believe their risk management systems are more effective than they actually are, exposing them to unanticipated risks. This can result in increased instances of fraud, regulatory non-compliance, financial losses, and damage to reputation.
5. Best practices for continuous monitoring and updating:
- Establish a routine for regular evaluation of model performance, including predefined metrics and benchmarks.
- Invest in training for staff to ensure they possess the latest skills in data analytics and risk management.
- Leverage advanced technologies, such as AI and machine learning, for more efficient and effective model updates.
- Create a flexible and adaptable risk management framework that can quickly integrate new data and insights.
The continuous monitoring and updating of risk models are akin to a navigational system in the ever-changing seas of financial risk. By paying attention to this aspect, financial institutions can ensure their risk assessment models remain accurate, relevant, and capable of effectively navigating the complexities of the financial world. This ongoing process is not just a regulatory requirement or a technical necessity; it is a strategic imperative that can significantly influence the overall health and resilience of a financial institution.
Causes of model drift in financial risk assessment
Model drift in financial risk assessment is an intricate issue that stems from various sources. Understanding these causes is crucial for financial institutions to effectively anticipate, identify, and mitigate the risks associated with model drift. Here’s an in-depth look at the primary causes:
1. Economic and market changes:
Financial markets and economies are dynamic entities, constantly influenced by global and local events, policy changes, and market trends. These changes can alter the financial behavior of customers and businesses, impacting the accuracy of existing risk models. For example, an economic downturn might lead to higher instances of loan defaults, which a model trained during a stable economic period might not accurately predict.
2. Evolving fraudulent techniques and behaviors:
Criminal activities, especially in the financial sector, are continuously evolving. As security measures improve, so do the techniques of those intent on circumventing them. A risk assessment model developed to identify certain types of fraud might become less effective as fraudsters adapt and develop new methods to evade detection.
3. Regulatory and compliance shifts:
The financial industry is heavily regulated, and changes in regulations can significantly impact risk assessment processes. When new regulations are introduced, or existing ones are modified, risk models may need to be adjusted to ensure compliance. Failing to do so can lead to regulatory penalties and increased legal risks.
4. Technological advancements:
The rapid pace of technological innovation can lead to changes in how financial transactions are conducted. New payment platforms, digital currencies, and online banking services can introduce new types of transactional data that existing models were not designed to handle. Moreover, advancements in data analytics and machine learning provide new tools and methodologies for risk assessment, which can render older models obsolete.
5. Changes in consumer behavior:
Consumer behavior in the financial sector can change due to various factors like demographic shifts, changes in consumer preferences, and the adoption of new financial products. These changes can affect spending patterns, borrowing habits, and investment strategies, which in turn impact the assumptions and parameters within risk assessment models.
6. Data quality and availability:
The quality and scope of data used to train risk models are crucial. Poor data quality or changes in data collection methodologies can lead to inaccurate model predictions. Additionally, the availability of new types of data (like social media or unstructured data) can provide new insights, necessitating model updates.
Understanding these causes of model drift is pivotal for financial institutions as they strive to maintain effective risk assessment models. Recognizing that model drift is not a sign of a flawed model but rather a reflection of the changing financial landscape is key. It underscores the need for continuous monitoring and updating of these models to ensure they remain effective tools for risk management in an ever-evolving financial environment.
The impact of ignoring model drift
Ignoring model drift in risk assessment within financial institutions can have far-reaching and sometimes severe consequences. Model drift, if left unchecked, can lead to a gradual but significant erosion of a model's effectiveness, impacting various facets of a financial institution’s operations. Here are some key impacts:
1. Reduced accuracy in fraud detection and risk prediction:
- As models become outdated, their ability to accurately detect fraud and assess risk diminishes. This can lead to an increase in fraudulent activities going undetected, or to misjudging the risk level of certain transactions or clients.
- The financial implications of such inaccuracies can be substantial, including significant financial losses and increased exposure to risky transactions or clients.
2. Increased false positives and negatives in transaction monitoring:
- An outdated model may either flag too many legitimate transactions as fraudulent (false positives) or fail to identify actual fraudulent transactions (false negatives).
- High rates of false positives can lead to operational inefficiencies and customer dissatisfaction due to unnecessary reviews and delays. Conversely, high rates of false negatives can mean actual risks are overlooked, leading to potential losses and regulatory scrutiny.
3. Regulatory compliance risks:
- Financial institutions are subject to stringent regulatory requirements, especially in areas like anti-money laundering (AML) and counter-terrorism financing.
- Models that are not up-to-date may fail to comply with new regulations, leading to legal repercussions, hefty fines, and sanctions from regulatory bodies.
4. Financial losses due to inadequate risk mitigation:
- Inaccurate risk models can lead to poor decision-making in credit assessment, investment strategies, and other financial activities.
- This can result in bad debts, unwise investments, or missed opportunities, directly impacting the financial health of the institution.
5. Damage to reputation and client trust:
- The inability to effectively manage risk can damage an institution's reputation. This is particularly true in cases of high-profile frauds or regulatory lapses that could have been prevented with accurate models.
- Loss of reputation can lead to a loss of client trust, which is a critical asset in the financial industry. This can have long-term impacts on customer retention and acquisition.
6. Strategic misalignment:
- Risk models often inform strategic decision-making. If these models are not reflecting the current environment accurately, it can lead to strategic plans that are misaligned with the actual risk landscape.
- This misalignment can result in ineffective resource allocation and missed opportunities for growth or innovation.
The impact of ignoring model drift is varied, affecting operational efficiency, compliance, financial performance, reputation, and strategic direction. The cumulative effect of these impacts underscores the importance of regularly monitoring and updating risk assessment models. It is not just about maintaining accuracy; it’s about preserving the institution's ability to function effectively and securely in a rapidly changing financial world. Addressing model drift is, therefore, a critical component of a robust risk management strategy.
Strategies to combat model drift

Combating model drift in financial risk assessment is a multifaceted challenge that requires a strategic and proactive approach. Financial institutions can implement various strategies to effectively manage and mitigate the risks associated with model drift. Here are some key strategies:
1. Regular model reassessment and updating:
- Conduct periodic reviews and reassessments of risk models to ensure they align with current data and risk environments. This involves not just tweaking the existing models but, if necessary, overhauling them to integrate new data and variables.
- Updating models could mean retraining them with recent data, incorporating new variables, or changing modeling techniques to better capture current risk dynamics.
2. Incorporating adaptive learning techniques:
- Utilize machine learning and artificial intelligence algorithms that are capable of adapting to new data patterns over time. These adaptive models can automatically adjust to changes in data, reducing the manual effort required for updates.
- Implement ensemble modeling approaches that use multiple models or algorithms to increase the robustness and adaptability of the risk assessment process.
3. Diversifying data sources:
- Expand the range of data sources used for modeling to include more diverse and comprehensive datasets. This could involve integrating alternative data sources like social media data, transactional metadata, or geospatial information.
- Regularly assess the quality and relevance of data sources, and be prepared to replace or supplement them as the financial landscape evolves.
4. Continuous monitoring and performance analysis:
- Establish continuous monitoring systems to track the performance of risk models in real-time or near-real-time. This helps in early detection of any signs of model drift.
- Use performance metrics and benchmarks to regularly evaluate the accuracy and efficiency of models. Key indicators might include false positive rates, detection rates, and predictive accuracy.
5. Staff training and development:
- Ensure that staff responsible for risk management and model development are trained in the latest techniques and trends in data analytics and risk assessment.
- Foster a culture of continuous learning and innovation, encouraging staff to stay informed about new methodologies and technologies in the field.
6. Scenario analysis and stress testing:
- Regularly conduct scenario analyses and stress tests to evaluate how risk models perform under various hypothetical conditions. This can help in understanding the potential impact of market changes or new types of financial crimes.
- Use the insights from these tests to refine and strengthen the models.
7. Collaboration and knowledge sharing:
- Engage in industry collaborations and forums to stay abreast of emerging risks, new modeling techniques, and regulatory changes.
- Share best practices and learnings within the institution and with industry peers to foster a more robust approach to managing model drift.
8. Leveraging regulatory and industry guidance:
- Stay informed about regulatory guidelines and industry standards related to risk modeling and compliance. Regulatory bodies often provide insights into expected practices and emerging risks.
- Incorporate regulatory feedback and industry benchmarks into the model development and updating process.
By employing these strategies, financial institutions can effectively combat model drift, ensuring their risk assessment models remain accurate, efficient, and aligned with the current risk environment. This proactive approach not only aids in maintaining compliance and operational efficiency but also supports the institution's strategic objectives by providing a reliable foundation for risk-based decision-making.
Advanced solutions for model management
In the ever-evolving landscape of financial risk assessment, staying ahead of model drift requires not just vigilance but also the adoption of advanced solutions for model management. These solutions leverage cutting-edge technologies and methodologies to enhance the accuracy, efficiency, and adaptability of risk models. Here's a deeper look into some of these advanced solutions:
1. AI and machine learning integration:
- Incorporating artificial intelligence (AI) and machine learning (ML) algorithms into risk models can significantly improve their predictive capabilities and adaptability. These technologies enable models to learn from new data continuously and adjust their predictions accordingly.
- Advanced ML techniques, such as deep learning and neural networks, can process and analyze complex, high-dimensional data more effectively than traditional statistical methods, providing a more nuanced understanding of risk factors.
2. Automated model monitoring and updating systems:
- Automated systems can be developed to monitor the performance of risk models in real-time and flag any signs of drift. These systems can also trigger automatic recalibrations or updates to models when certain thresholds are met, ensuring continuous alignment with current data trends.
- Such automation reduces the manual burden on staff and allows for more rapid adjustments to models, enhancing responsiveness to changing risk landscapes.
3. Data quality management tools:
- Implementing robust data quality management tools is critical for ensuring that the data feeding into risk models is accurate, complete, and relevant.
- Tools that can clean, validate, and standardize data inputs play a crucial role in maintaining the integrity of risk models, as poor-quality data is a major contributor to model drift.
4. Scenario analysis and predictive analytics:
- Advanced scenario analysis tools can simulate a wide range of risk scenarios, including extreme and rare events, to test the resilience of risk models under various conditions.
- Predictive analytics can forecast future trends and risk patterns, allowing financial institutions to proactively adjust their models to anticipate these changes.
5. Cloud computing and big data technologies:
- Leveraging cloud computing provides scalability and flexibility in managing large volumes of data and complex modeling processes. It also facilitates easier integration of new data sources and technologies.
- Big data technologies enable the processing and analysis of vast datasets that are increasingly common in financial risk assessment, allowing for more comprehensive risk models.
6. Collaborative platforms for model development:
- Developing risk models can be enhanced through collaborative platforms that allow data scientists, risk analysts, and other stakeholders to work together seamlessly.
- These platforms can facilitate sharing of insights, models, and best practices across different teams and departments, fostering a more integrated approach to risk management.
7. Regulatory technology (regtech) solutions:
- Regtech solutions can be utilized to ensure that risk models comply with regulatory requirements and standards. These solutions often include features for regulatory reporting, compliance tracking, and audit trails.
- By integrating regulatory considerations directly into model management processes, financial institutions can better align their risk assessment practices with current regulatory expectations.
Adopting these advanced solutions for model management allows financial institutions to not only combat model drift but also to stay ahead in a rapidly changing risk environment. These technologies and tools provide a more dynamic, responsive, and efficient approach to risk model management, ultimately supporting better decision-making and enhancing the overall risk posture of the institution.
The future of risk assessment
The future of risk assessment in financial institutions is poised to be shaped by a confluence of technological advancements, evolving regulatory landscapes, and changing market dynamics. As financial institutions grapple with the challenges of model drift and the need for more accurate and dynamic risk assessment models, several key trends and developments are emerging. These will not only redefine how risk is assessed but also how it is managed and mitigated.
1. Increased reliance on artificial intelligence and machine learning:
- AI and ML will become even more integral to risk assessment. These technologies offer the ability to process vast amounts of data, learn from new information, and adapt to changing patterns more efficiently than traditional models.
- Future risk models will likely leverage advanced AI to perform predictive analytics, identify emerging risks, and provide more nuanced insights into potential threats.
2. Integration of big data and alternative data sources:
- The use of big data in risk assessment is expected to grow, allowing for a more comprehensive analysis of risk factors. This includes not just financial data but also alternative data sources such as social media, behavioral analytics, and geospatial data.
- The challenge will be to effectively integrate and analyze these diverse data streams to enhance the accuracy and breadth of risk assessment.
3. Advancements in real-time risk assessment:
- The ability to assess risk in real-time, or near-real-time, will become increasingly important. This capability will allow financial institutions to respond more quickly to emerging threats and adapt their strategies on the fly.
- Real-time risk assessment will be particularly crucial in areas like fraud detection and AML monitoring, where timely intervention can prevent significant losses.
4. Greater emphasis on cybersecurity risk:
- As financial services become increasingly digitized, cybersecurity risks will become a more prominent part of overall risk assessment strategies.
- Future risk models will need to incorporate cybersecurity parameters and be adept at identifying and mitigating threats in the digital domain.
5. Regulatory technology for compliance:
- Regtech solutions will play a key role in ensuring compliance with evolving regulatory requirements. These technologies will help institutions navigate complex regulatory landscapes and integrate compliance more seamlessly into risk management processes.
- Automation and AI in regtech will also aid in more efficient reporting, monitoring, and compliance analysis.
6. Collaborative and integrated risk management:
- The future will see a more collaborative approach to risk management, with integration across different departments and functions within financial institutions.
- This integrated approach will facilitate a more holistic view of risks, combining insights from various areas like finance, operations, compliance, and IT.
7. Enhanced focus on ethical and responsible AI:
- As AI becomes more prevalent in risk assessment, there will be an increased focus on ensuring these models are ethical, transparent, and free from biases.
- Ensuring the responsible use of AI will be crucial to maintain trust and integrity in risk assessment processes.
8. Personalization and customer-centric risk models:
Future risk models may become more personalized, taking into account individual customer behaviors and preferences. This could lead to more accurate risk assessments and more tailored financial products and services.
The future of risk assessment in financial institutions will be characterized by rapid technological innovation, increased data integration, real-time analytics, and a more holistic and proactive approach to managing risk. These advancements will enable financial institutions to not only respond more effectively to existing risks but also to anticipate and mitigate emerging ones, thereby ensuring greater financial stability and security in an increasingly complex and interconnected world.
Conclusion
As we reflect on the intricate dynamics of model drift in risk assessment, it's important to connect this understanding with broader themes in financial risk management, similar to those discussed in our previous article, "Strategies to Ensure AML Data Integrity." Both topics emphasize the necessity for constant vigilance and adaptability in an ever-evolving financial landscape.
The key takeaway is the critical role of proactive strategies in maintaining the integrity and effectiveness of risk assessment models. This approach aligns with ensuring data integrity in AML strategies, highlighting a consistent theme: in the face of changing financial environments, the need for dynamic and responsive risk management is paramount. Staying ahead of these changes is not just a matter of regulatory compliance but a cornerstone of maintaining financial security and operational resilience.